Towards a “universal translator” for neural dynamics at single-cell, single-spike resolution
- 1Columbia University
- 2Stanford University
- 3Universitat Politècnica de Catalunya
- 4Georgia Institute of Technology
- 5Mila
- 6McGill University
- 7Champalimaud Foundation
- 8The International Brain Laboratory
Overview
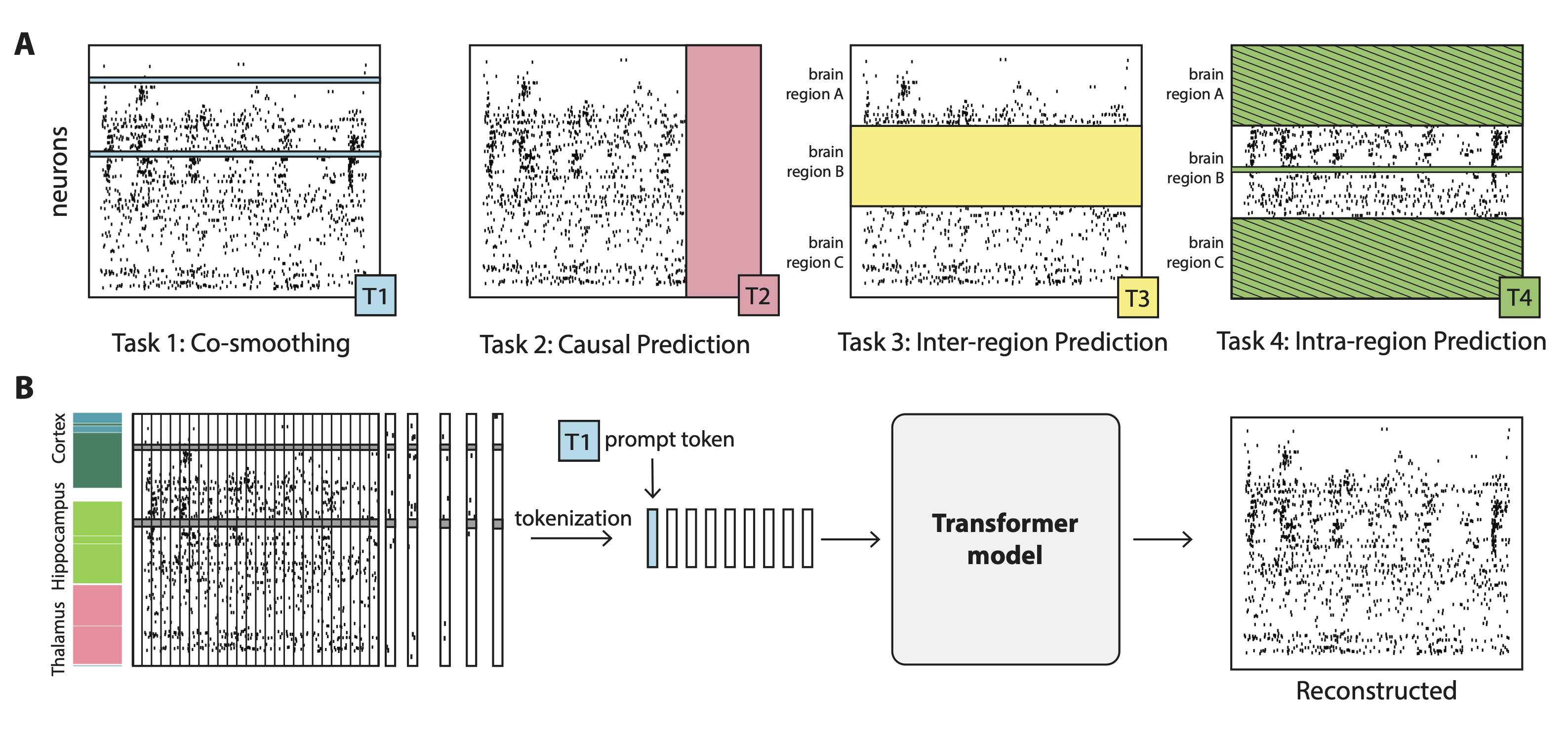
Abstract
Neuroscience research has made immense progress over the last decade, but our understanding of the brain remains fragmented and piecemeal: the dream of probing an arbitrary brain region and automatically reading out the information encoded in its neural activity remains out of reach. In this work, we build towards a first foundation model for neural spiking data that can solve a diverse set of tasks across multiple brain areas. We introduce a novel self-supervised modeling approach for population activity in which the model alternates between masking out and reconstructing neural activity across different time steps, neurons, and brain regions. To evaluate our approach, we design unsupervised and supervised prediction tasks using the International Brain Laboratory repeated site dataset, which is comprised of Neuropixels recordings targeting the same brain locations across 48 animals and experimental sessions. The prediction tasks include single-neuron and region-level activity prediction, forward prediction, and behavior decoding. We demonstrate that our multi-task-masking (MtM) approach significantly improves the performance of current state-of-the-art population models and enables multi-task learning. We also show that by training on multiple animals, we can improve the generalization ability of the model to unseen animals, paving the way for a foundation model of the brain at single-cell, single-spike resolution.
Highlights
- A novel multi-task-masking (MtM) approach which can be applied to multi-region datasets to successfully learn representations that lead to better downstream task performance.
- A prompt-based approach for test-time adaptation which improves performance on a variety of downstream tasks during inference.
- Scaling results that demonstrate that having data from more animals provides benefits on held-out animals and sessions as well as on unseen tasks.
- A new multi-task, multi-region benchmark for evaluating foundation models of neural population activity.
Single-session
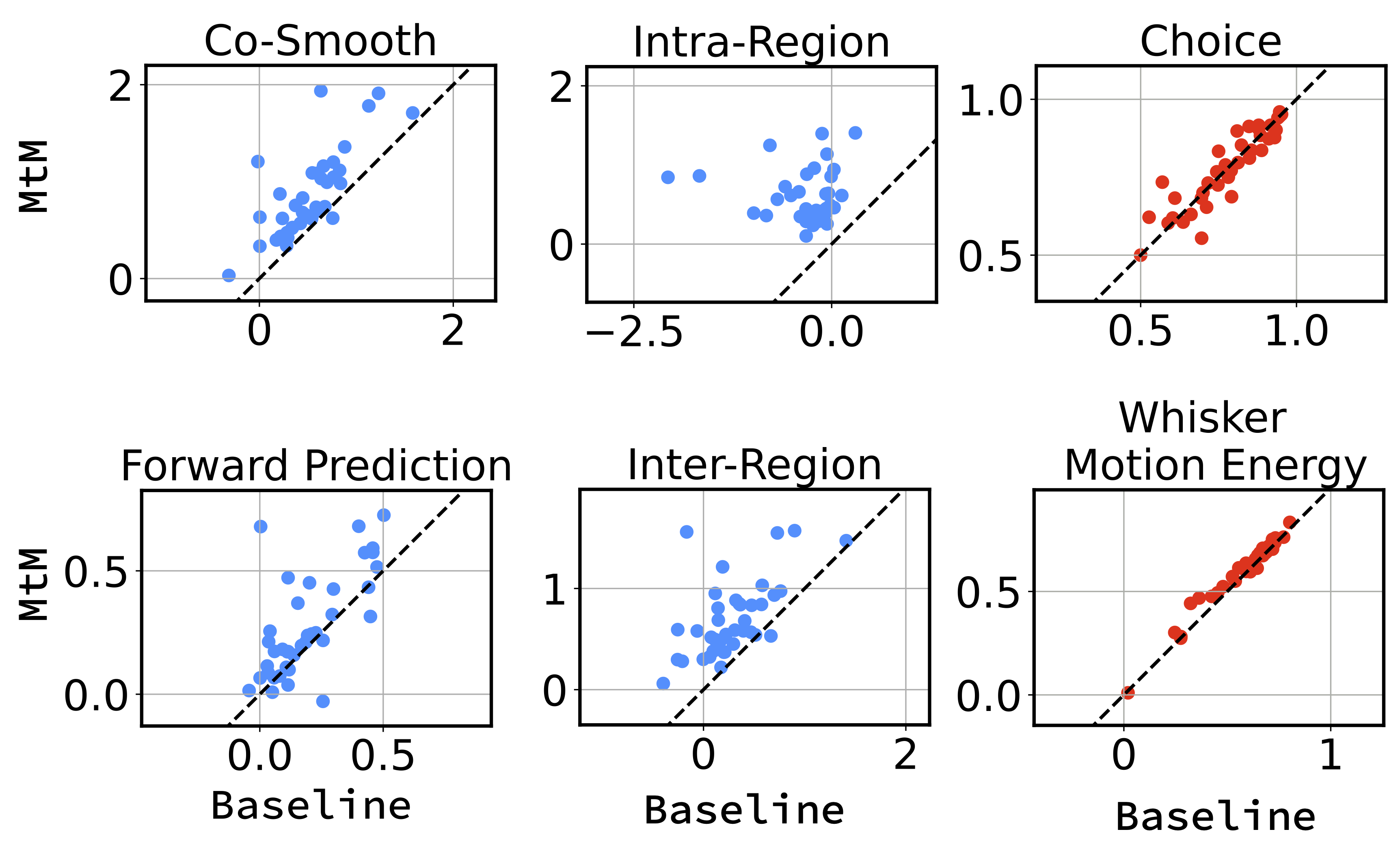
Comparison of the temporal masking baseline and the proposed MtM method for NDT1 on activity reconstruction and behavior decoding across 39 sessions. Each point represents one session. For activity reconstruction, we report the average bps. For choice and whisker motion energy decoding, we report the average accuracy and R2, respectively, across all test trials.
Multi-session
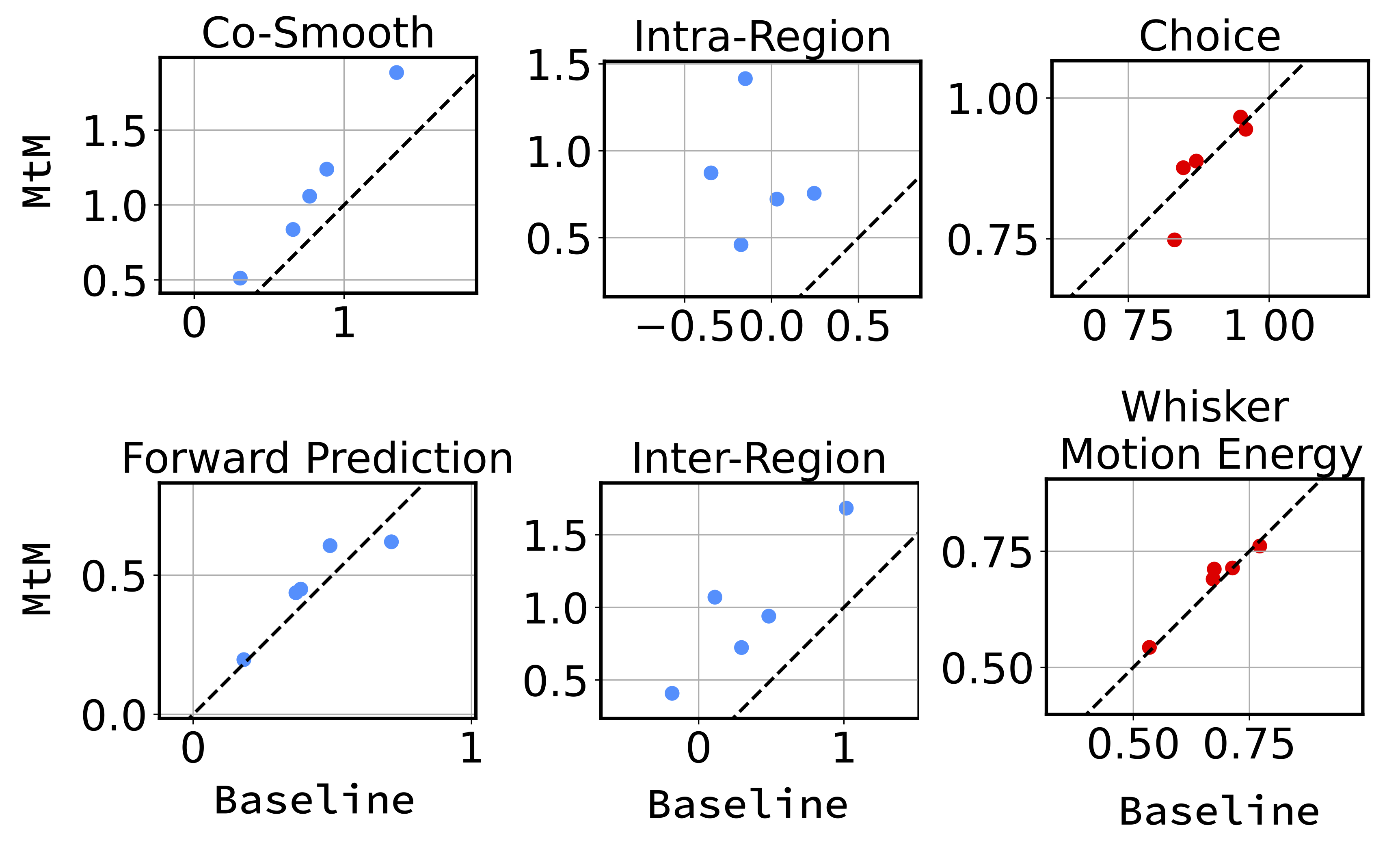
Fine-tuning performance comparison of NDT1-stitch pretrained with MtM vs. temporal masking for activity reconstruction and behavior decoding across 5 held-out sessions. For activity reconstruction, each point shows the average bps across all neurons in a held-out session. For choice and whisker motion energy decoding, each point represents the average accuracy and R2, respectively, across all test trials in one session.
Scale analysis
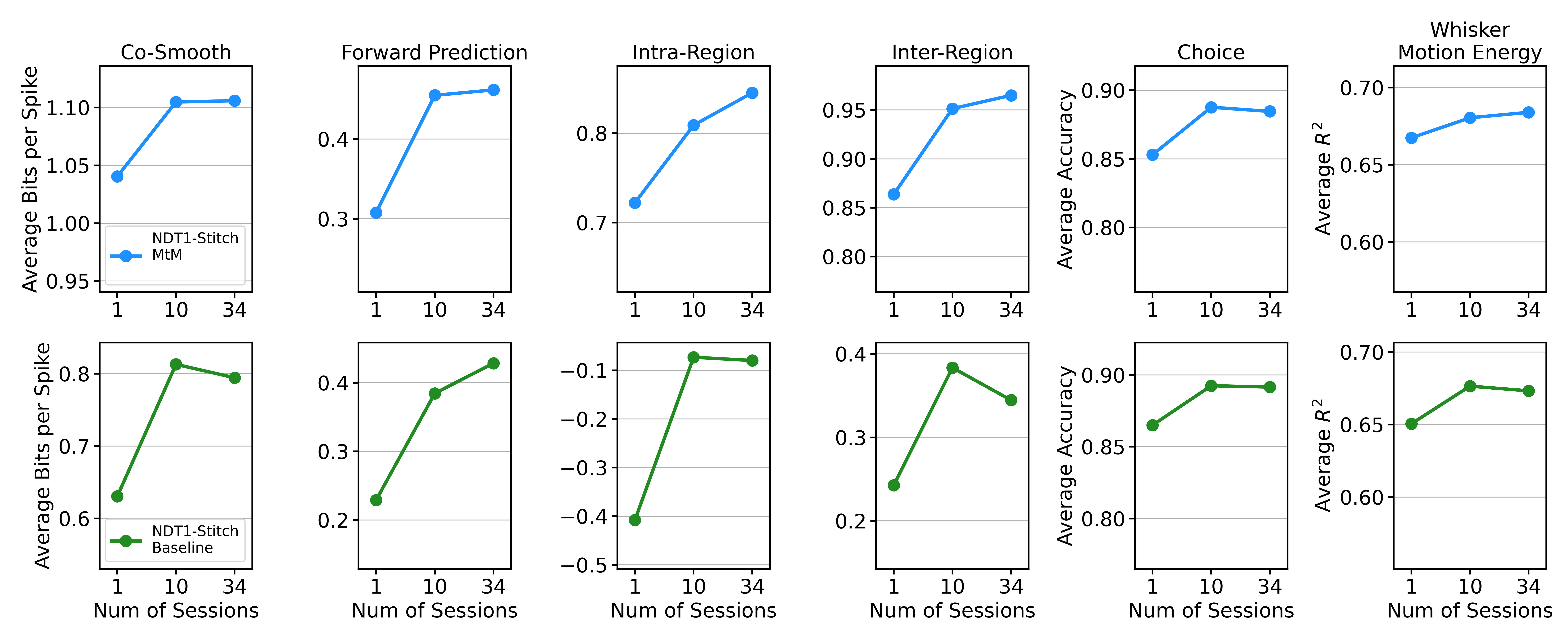
Comparison of scaling curves between NDT1-stitch pretrained with the MtM method vs. the temporal masking baseline. The reported metrics - neuron-averaged bits per spike (bps), choice decoding accuracy, and whisker motion energy decoding R2 - are averaged over all 5 held-out sessions. We fine-tune each pretrained model with its self-supervised loss (MtM or temporal) on the 5-heldout sessions and then evaluate with all of our metrics. "Num of Sessions" denotes the number of sessions used for pretraining.
Behavior decoding from individual brain regions
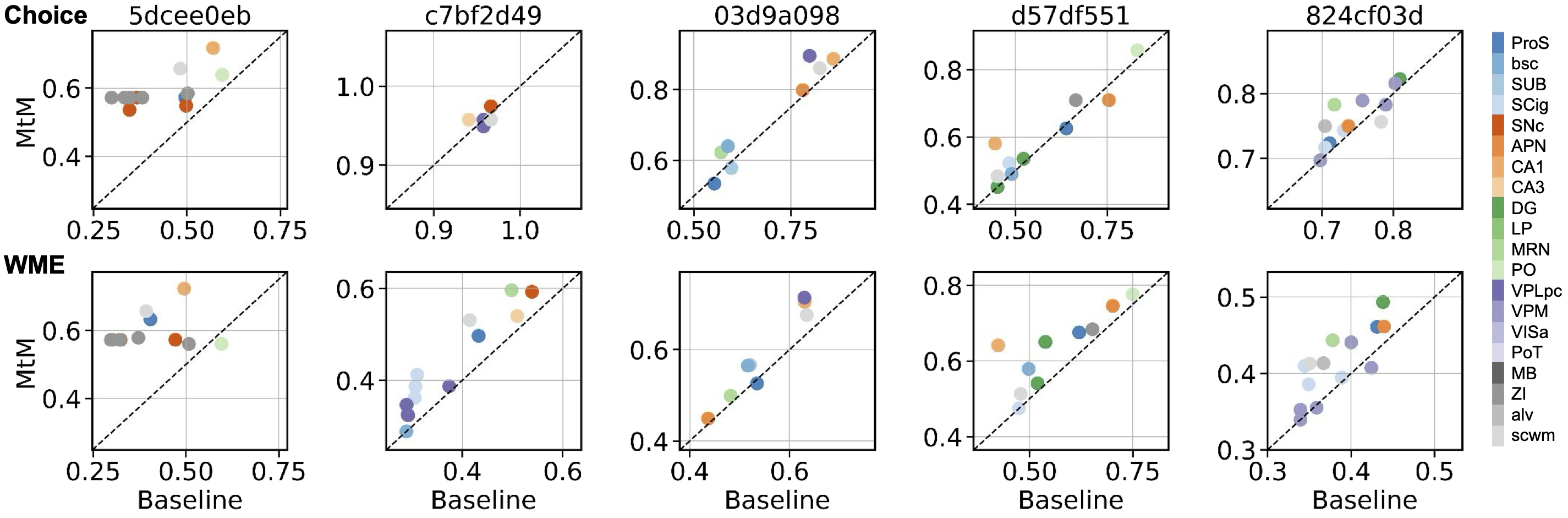
Comparison of NDT1-stitch pretrained with the MtM method vs. the baseline temporal masking on behavior decoding from individual brain regions. The rows display choice decoding accuracy and whisker motion energy decoding R2. Columns represent individual held-out sessions. Each point shows the behavior decoding performance when using neural activity from a specific brain region, with colors denoting different brain regions.
Single neuron evaluation
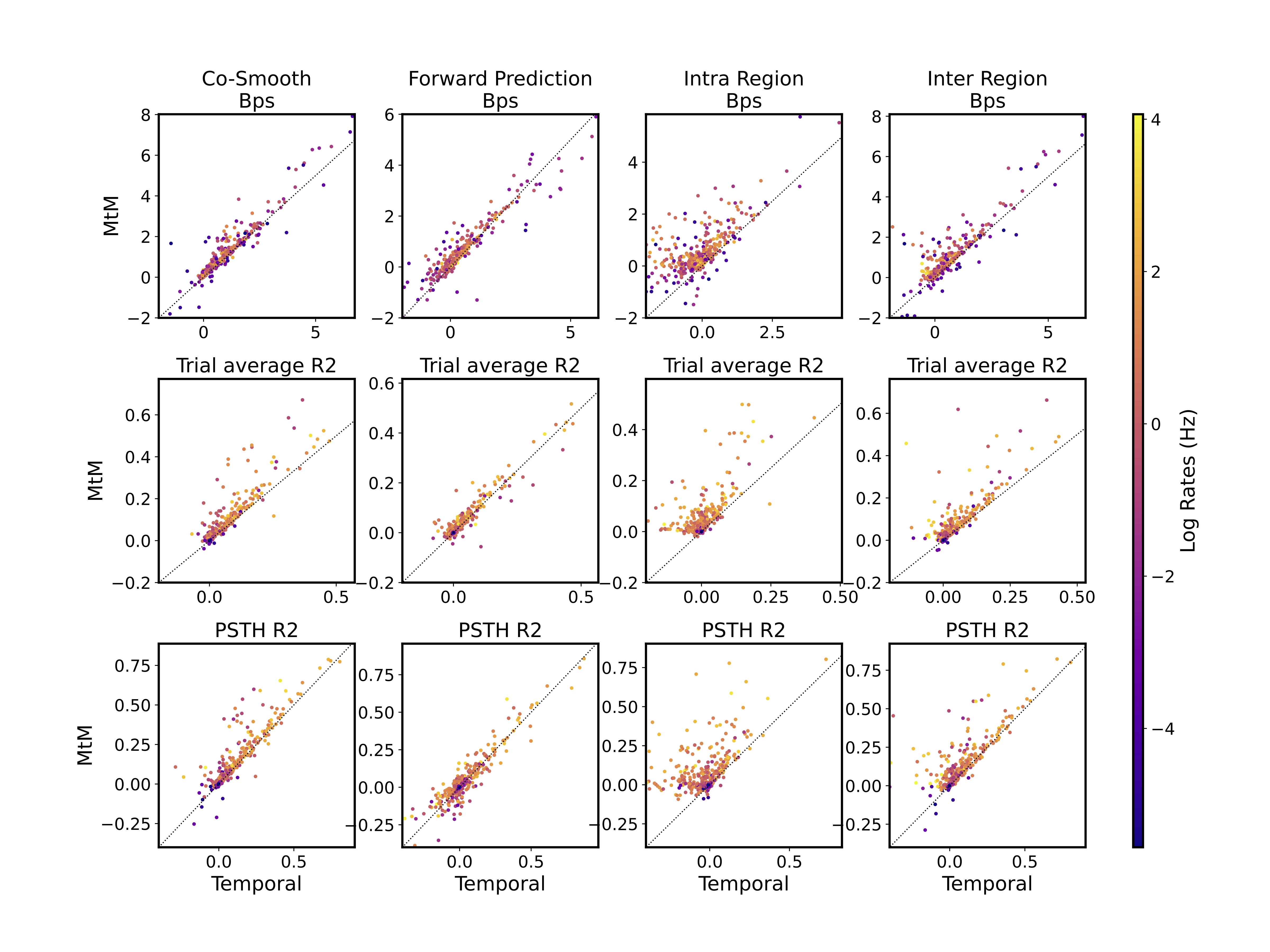
Single neuron activity reconstruction analysis for NDT1 in one session. To evaluate the reconstruction quality for each neuron, multiple metrics are computed: Bits per spike (Bps), R2 between the ground truth and predicted peristimulus time histogram (PSTH R2), and the single-trial R2 averaged across all trials (Trial average R2). Each point represents one neuron, with the color indicating the neuron's log firing rates in Hertz (Hz).
BibTeX
If you find our data or project useful in your research, please cite:@InProceedings{Zhang_2024_arXiv, author = {Zhang, Yizi and Wang, Yanchen and Benetó, Donato Jiménez and Wang, Zixuan and Azabou, Mehdi and Richards, Blake and Winter, Olivier and The International Brain Laboratory and Dyer, Eva and Paninski, Liam and Hurwitz, Cole}, title = {Towards a “universal translator” for neural dynamics at single-cell, single-spike resolution}, booktitle = {arXiv}, month = {July}, year = {2024}, url = {http://arxiv.org/abs/2407.14668} }